Jonathan S. Miller, Michael Gunnels, Fridon Shubitidze
White River Technologies, Inc., Lebanon, N.H.
Abstract
The effectiveness of advanced Electromagnetic Induction (EMI) sensor technologies to discriminate potentially hazardous Unexploded Ordnance (UXO) or other Munitions and Explosives of Concern (MEC) from non-hazardous metal debris has been established through the implementation of the US Department of Defense (DOD) Advanced Geophysical Classification Accreditation Program (DAGCAP) and the associated international ISO/IEC 17025 standard. A central principle of the DAGCAP program is the application of a blind seeding process on all Advanced Geophysical Classification (AGC) projects. To verify the AGC technology used on a project has detected and correctly classified all MEC, inert or proxy items are buried in undisclosed locations throughout the site to test the AGC process. To meet DAGCAP standards, the contracted Geophysical Classification Organization (GCO) performing AGC must classify 100 percent of these blind seeds or perform a Root Cause Analysis (RCA) for those that are not. While blind seeding is an effective process for ensuring the integrity of the classification decision, it can be costly, particularly for underwater UXO sites, due to the time required to bury and accurately survey a large sample of seeds throughout a site. A cost-effective way to increase the size of the seeding program is to use synthetic seeds, which incorporate the modeled signal from seed items into the EMI survey data acquired at the site. This method can significantly increase the number of seeds to better establish the operating envelope of the AGC technology given site-specific conditions such as environmental noise, terrain, and geology.
Introduction
One could make the case that blind seeding is the keystone of the DAGCAP program. It is a way to ensure that the AGC technology is performing as expected on a project. Because many Munitions Response (MR) sites contain a relatively small percentage of MEC compared to non-hazardous items, it can be difficult to ascertain the effectiveness of AGC decisions with intrusive investigation of native objects alone. By emplacing seeds at or near the maximum operating depth, seeding not only guarantees a statistically relevant sample size, but also demonstrates the technology is achieving the expected operating envelope. For these reasons, blind seeding was a critical component for achieving regulator acceptance of AGC technology during the inception of the DAGCAP program.
Blind seeding is implemented through two programs on AGC projects. The Quality Assurance (QA) program is performed by either the Government or a third-party contractor. These “validation” seeds are designed to verify that the GCO is implementing their quality system correctly. The quality system defines a set of standard operating procedures that guide all phases of work from site preparation to data collection to data processing to intrusive investigation. Validation seeds are emplaced at sub-maximal depths to demonstrate that if the GCO follows these procedures correctly, they are guaranteed to identify the seeds correctly.
The Quality Control (QC) program is implemented directly by the GCO contractor through the means of an internal firewall that segments the seeding team from the rest of the project team. QC seeds are designed to test both the GCO’s quality system and the AGC technology operating envelope. Accordingly, QC seeds are buried at various depths throughout the vertical boundary of the site, including seeds placed at or near their maximum expected depth of classification.
Effective QA can be accomplished with a relatively small number of validation seeds. Because these seeds are not intended to be as challenging nor to test the AGC technology under all conditions encountered at a site, validation seeds need only be placed at a rate equivalent to one seed encountered per survey team per day. QC, on the other hand, would benefit significantly from a larger number that tests the technology throughout the range of conditions that may be encountered on a site. Because environmental factors such as noise (e.g., powerlines), terrain, and geology can vary considerably throughout a site, verifying the AGC system operating envelope with all these variables may require a much larger distribution of QC seeds.
Synthetic Seeding
One way to boost seeding sample size without the cost associated with burying and surveying seeds throughout a site is to use synthetic seeds. Synthetic seeding is accomplished by combining real survey data with synthetically generated signals from seed objects using electromagnetic dipole-based models. To fully understand this approach, we must first consider the 3D electromagnetic sensor concept.
Advanced 3D EMI sensors incorporate an array of multi-axis transmitters and receivers to provide high spatial and temporal resolution of anomalies created by buried metal objects. This combination of multi-directional transmit and receive elements produces a spatially and temporally rich data set that contains information about how the subsurface object responds to the transmitter magnetic fields in both space and time. Specifically, the transmitter primary magnetic fields induce eddy currents within a target. These induced eddy currents generate a target response in the form of a secondary, time-varying magnetic field. As the transmitter fields impinge on the object in different directions, the target responses can be represented by the magnetic polarizations along the object’s principal axes (i.e., one large longitudinal and two smaller lateral for axially symmetric objects such as UXO). The responses of these principal axes to the impinging magnetic fields are known as the time-dependent electromagnetic polarizabilities of the object (Figure 1) and they are used to characterize physical attributes such as diameter, wall thickness, aspect ratio, and material composition.
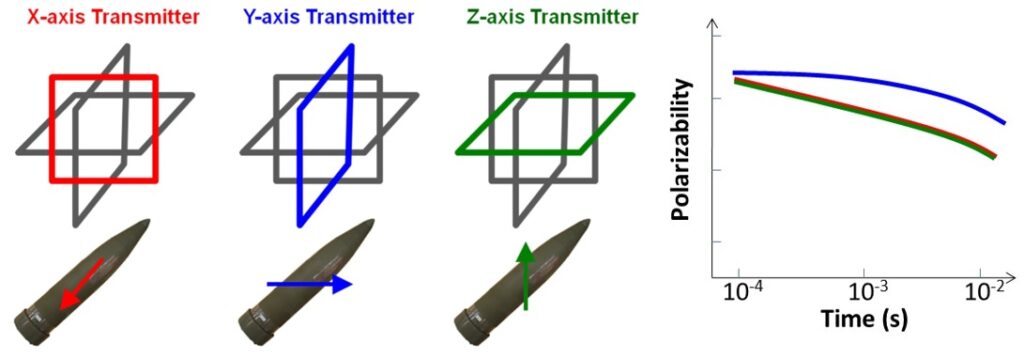
Figure 1. LEFT: Each transmitter creates a unique polarization of the buried metal object that is determined by the direction of the magnetic field impinging on it. RIGHT: The overall response of the object to the transmitters can be deconstructed into principal components described by a set of three time-dependent principal electromagnetic polarizabilities.
Much as the principal electromagnetic polarizabilities of an object can be derived from the object’s response signal recorded in survey data, the reverse is also true. The electromagnetic forward model used to derive the polarizabilities from inversion of the data can also be applied to derive the signals generated by the object from the polarizabilities. This process is the basis for synthetic seeding.
Determining the Operating Envelope
As part of DAGCAP, the DoD has catalogued an extensive library of polarizability signatures derived from several hundred different munitions commonly found on current or former DoD installations. These library polarizabilities can be used to generate site-specific data driven synthetic data corresponding to any item in the library catalog. As an example of this process, we can consider the case of a 37mm projectile signature. Using the library polarizabilities for this item, we can effectively synthetically seed a site with a large sample size of 37mm projectiles placed virtually at a range of depths, locations, and orientations.
Figure 2 presents a grid of dynamic AGC sensor data comprising overlapping transects. Initially, we designate positions, depths, and orientations for our synthetic 37mm seeds within a global coordinate system on the grid. Subsequently, in the immediate vicinity of each synthetic seed location, we use the sensor’s positions and orientations to compute the primary magnetic field at the synthetic seed’s location. We then determine the induced magnetic dipole using library polarizabilities. We calculate the induced voltage at the sensor receivers for each transmitter coil. This process is performed at each sensor location to generate a signal based on the library polarizabilities that is contingent upon the seed’s location and orientation with respect to the sensor. Finally, the signals generated from the library polarizabilities are added to the raw data. We then reprocess the synthetically seeded data files using the standard classification workflow. In this manner, we capture the effects of site-specific factors such as environmental noise and geologic response on the classification results. The classification workflow applies project-specific processing procedures to the synthetically seed data, verifying appropriate selection of data processing parameters.
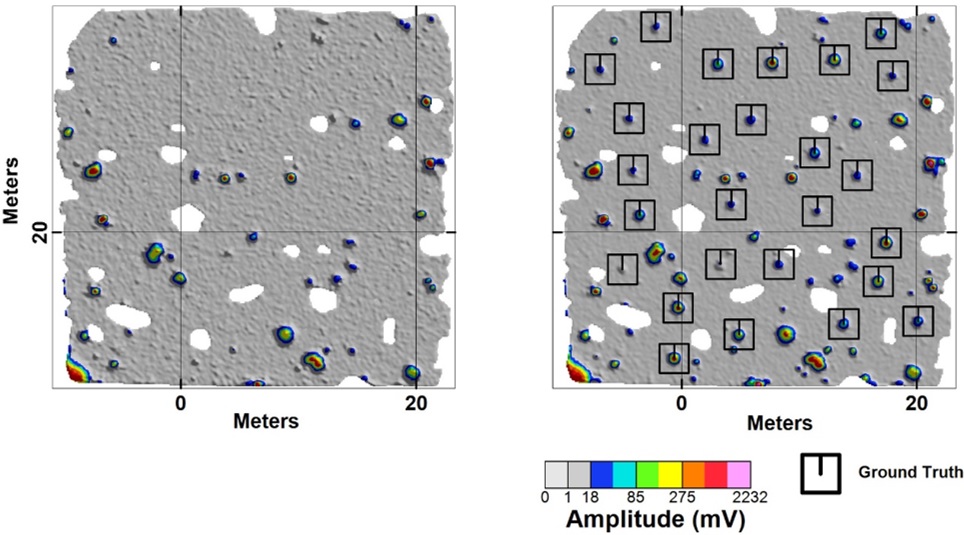
Figure 2. LEFT: Gridded dynamic AGC survey data acquired at a site. RIGHT: Grid map after synthetic 37mm projectile seeds (black squares) are emplaced. Seeds range in depth from 15 cm to 60 cm.
After inverting the seeded data, we can compare the polarizabilities for each seed to the library from which the seed signature was derived. A library match statistic is a value between 0 and 1 that indicates how closely a set of polarizabilities matches a library signature. Values close to 0 indicate a low confidence that the item is the library target while values close to 1 indicate a high confidence that the item is the library target. Typically, a confident classification decision can be made that the item is the library target when the match statistic is above a range of 0.8 – 0.9. A value in this range is selected per project as the target selection threshold. Targets above this threshold are selected for intrusive investigation and targets below this threshold are rejected as clutter. Library match values above this threshold indicate that the item is classified as the library target while values below this threshold indicate the item is not classified as the library target. Using a target selection threshold, we can determine whether the seed items can be correctly classified as the library target (37mm projectile) with a high confidence. This library match process quantifies the effects of environmental factors on classification of the seed by demonstrating the conditions in which the seed is no longer classifiable (i.e., it produces a library match below the threshold). Figure 3 shows an example of a synthetic 37mm seed library match.
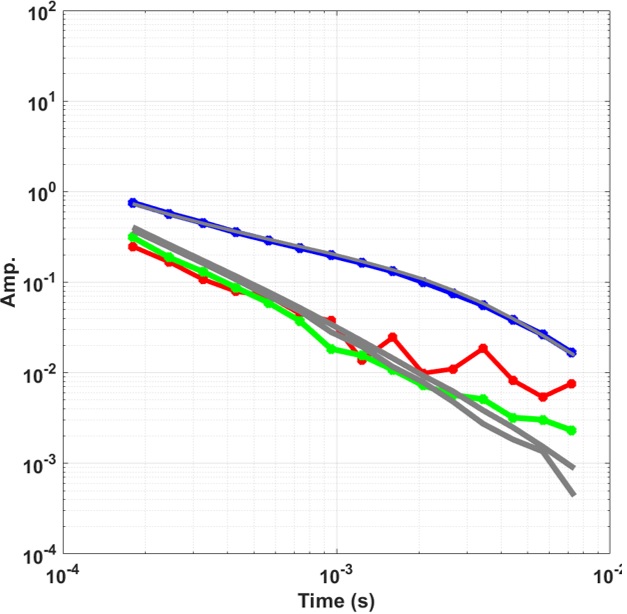
Figure 3. Library match showing the polarizabilities obtained from a synthetic 37mm seed (blue, red, and green lines) compared to the library polarizabilities (grey lines) for this item.
Using the library match analysis, synthetic seeding provides confirmation that the operating envelope (i.e., required classification depth for a specific target of interest) can be achieved under the variety of conditions that may be encountered at a site. Conditions that may affect the depth of classification include site geology (i.e., elevated background response), environmental noise (e.g., power lines), terrain-induced noise, or saturated response areas (i.e., localized high target density regions).
Site-Specific Factors
Synthetic seeding is particularly useful for identifying site-specific factors that may limit the ability to achieve the planned operating envelope and for deriving data driven classification performance statistics. Physical seeding may not effectively capture the effect of environmental variability on AGC performance due to a limited sample size. The larger number afforded by synthetic seeding is more likely to capture corner-case scenarios (i.e., scenarios in which overlapping conditions such as high target density and elevated background combine to limit operating performance). Synthetic seeding can be a tool that not only identifies these conditions, but also provides a means for testing and verifying remedies to these challenges.
An example of this verification process is demonstrated using powerline noise interference. Powerlines and nearby low frequency, high power communication systems commonly interfere with EMI sensors by elevating background noise levels. Noise levels can vary significantly depending on the powerline height, depth, or time of day (load on the lines). Several filtering methods are available to reduce the effect of powerline noise, and results can be evaluated to ensure effective noise reduction without effect to detection and classification.
Figure 4 shows an example of powerline noise interference and the implementation of a filter to reduce these effects. In this case, we applied synthetic seeds to the data to evaluate the performance of the filter for improving classification in these conditions. We synthetically seeded the raw data with a distribution of 37mm projectiles and determined the library match for each seed after applying both the standard classification workflow and a site-specific workflow that implemented a powerline filter. We compared the classification results from the standard approach to the filtered approach and determined that the filter significantly improved the operating envelope for this target of interest.
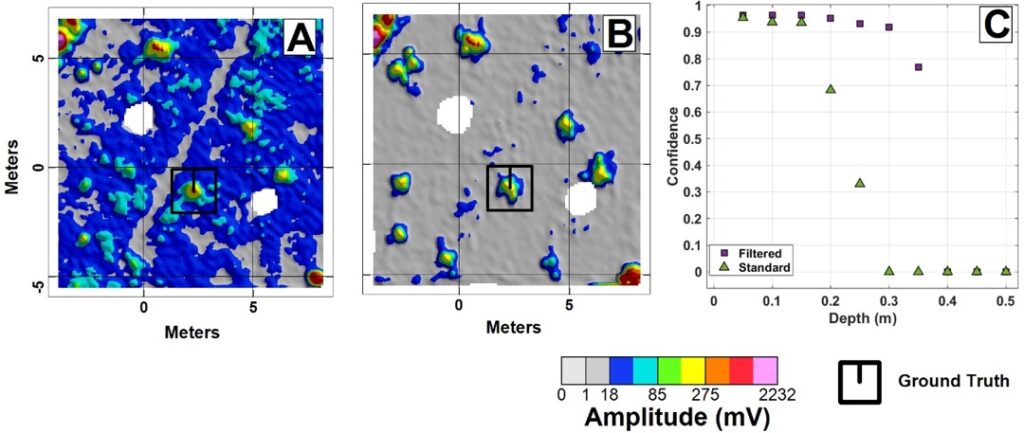
Figure 4. Example of powerline interference. A: Map showing the presence of elevated background noise due to a powerline running southwest to northeast through the grid. The map shows the gridded vertical component data. Due to the rotational direction of the powerline field, the vertical component data show elevated noise near the powerline, but not directly underneath because the noise there is almost entirely oriented in the horizontal plane. Ground truth for a seed location is shown as the black square. B: Noise levels are significantly reduced by applying a powerline noise filter. Note the seed’s anomaly now produces a much higher signal-to-noise ratio. C: Classification confidence based on library match for synthetic seeds processed using the standard (triangles) and filtered (squares) methods. Without the filter, the seeds produce a high confidence match to a depth of only 0.15 m. Applying the filter, the high confidence match depth is extended to 0.30 m. A confidence value of 0 means that the seed signal is indistinguishable from noise.
Another challenge at many sites is the presence of magnetic geology that can produce an elevated and spatially variable background response. Separating the background response from the target response is a critical step in effective AGC. Without correct identification and removal of the background response, the background response may get mixed with the target signal and result in incorrect classification of a target. Background identification and removal in AGC is typically performed using two methods: 1) static removal where the background response from one location is subtracted from other sensor data across the site (applies to both static and dynamic sensor data); and 2) dynamic removal where background response is determined from a highly localized region within the site and subtracted from sensor response within that region (applies only to dynamic sensor data).
Static removal is effective when the background response is relatively constant throughout the site; however, this method can be problematic when background response is high and highly variable as is commonly the case at sites with magnetic geology. In these conditions, the dynamic background removal approach may be more effective. As the dynamic background response window is applied to different regions throughout the site, the background is constantly updated to reflect the local response. A window length is selected based on the characteristics of the site. Longer windows are closer to static removal in that they apply one background correction to a larger area. A long window may be desirable for sites with little background variability. Shorter windows may be better suited for sites with high background variability because they are more sensitive to background changes over a smaller area. The potential drawback of a shorter window is the possibility of including a greater proportion of target signal with background response and, therefore, incorrectly over-leveling the data.
Synthetic seeds can be used to verify the optimal selection of a background removal approach for a site. For example, proper implementation of synthetic seeds can confirm the background removal window length effectively removes the background without removing target signals resulting in misclassification. Consider the data presented in Figure 5. The maps show a region of elevated background response due to magnetic geology. Each map displays the effect of applying a different length background leveling window to the data. Prior to performing the background leveling, synthetic seeds are placed at a range of depths in the elevated background region. Performing classification on the leveled data allows us to determine the effect of window length on the maximum operating depth.
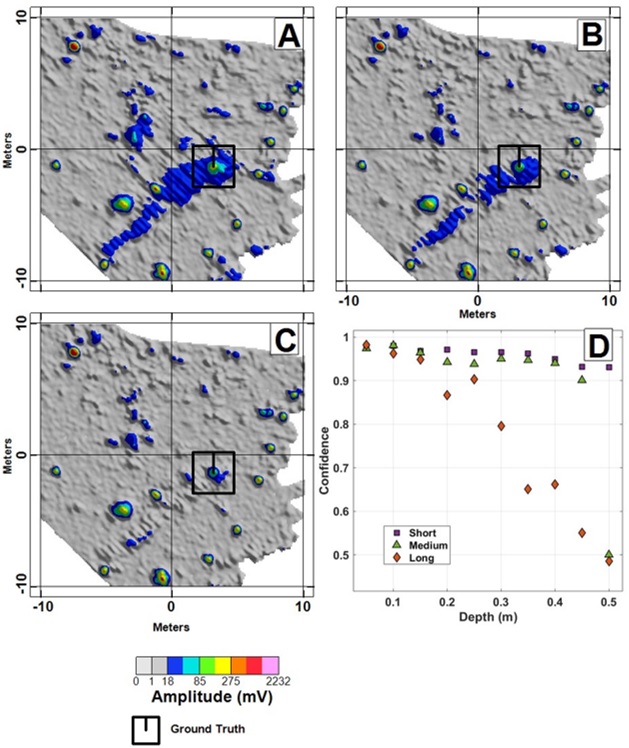
Figure 5. Data corresponding to a 37mm projectile synthetic seed buried at a range of depths at a site with elevated background response. The location of the synthetic seed is shown with the black square on each map. A: Gridded data leveled using a “long” filter length. Applying this window length is similar to performing static background leveling. B: Gridded data leveled using a “medium” filter length. C: Gridded data leveled using a “short” filter length. Note that as the filter window length decreases, the background response (blue region in center of map) decreases, and the seed anomaly becomes more distinct. D: Classification results corresponding to the three filter window lengths. The long filter (diamonds) provides high confidence classification to a depth of only 0.25 m, while the medium (triangles) and short (squares) filter lengths provide high confidence classification to depths of 0.45 m and 0.50 m, respectively.
Conclusions and Recommendations
Data driven synthetic seeding is an effective tool for supplementing QC measures on AGC projects. It provides a cost-effective method to capture the effects of site-specific factors on data quality, and to quantify and verify the performance of processing techniques used to mitigate these effects. Additionally, synthetic seeding can be used to generate extensive site-specific datasets for post-AGC assessment purposes.
While synthetic seeding captures many of the attributes of physical seeding, there are certain aspects of AGC that are better evaluated by emplacement of physical seeds. For example, it is possible to acquire AGC data with positional errors that would not be identified with synthetic seeding. Therefore, synthetic seeding is best suited as a method to augment and not replace the physical seeding program.
Acknowledgements
Thanks to HGL, Parsons, Tetra Tech, and Weston for providing sample data sets used for synthetic seeding.
Author Bios
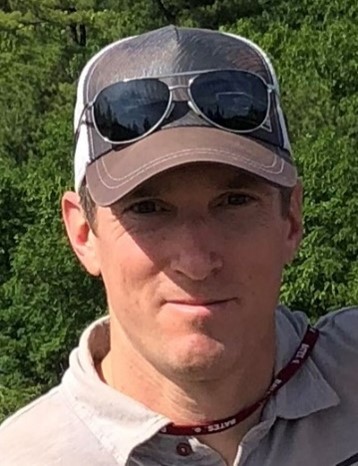
Jonathan Miller is a Senior Engineer at White River Technologies. He obtained a B.S. degree in Physics from Bates College and an M.S. degree in Mechanical Engineering from the University of Vermont. His research interests include application of magnetic and electromagnetic sensing to detection, subsurface characterization, and infrastructure monitoring. He is currently performing several projects involving integration of these sensor modalities with a variety of platforms.
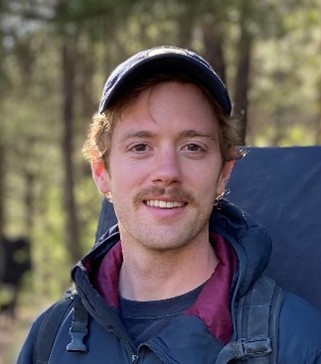
Michael Gunnels is an R&D Geophysicist at White River Technologies. He obtained a B.S. degree in Geology from Central Michigan University and an M.S. degree in Geophysics from the University of Missouri. His interests include efficient processing of magnetic and electromagnetic data, algorithm development / testing, and data fusion / visualization. He is currently working on algorithm development and assisting in commercial EM processing work.
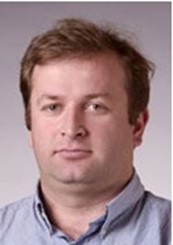
Fridon Shubitidze is a Senior Research Physicist at White River Technologies and a Research Professor with the Thayer School of Engineering, Dartmouth College, Hanover, NH. He received the degree of Diploma radio physicist (M.S.) from the Sukhumi branch of Tbilisi State University (TSU), Sukhumi, Republic of Georgia, in 1994 and the Cand.Sci. (Ph.D.) degree in radio physics (applied electromagnetics) from TSU in 1997. His current work interests focus on development of advanced EM sensing technologies (from 0 Hz up to GHz) for detecting and classifying buried explosive hazards on land and in underwater environments. In 2011, Dr. Shubitidze and his group received the Munitions Response Project-of-the-Year Award given by the Strategic Environmental Research and Development Program for developing and demonstrating advanced EMI models and algorithms for UXO detection and classification.